Search QIN

University of Michigan (team#2)
Biomarkers for Staging and Treatment Response Monitoring of Bladder Cancer
Lubomir Hadjiyski, PhD
lhadjisk@med.umich.edu
Grant Number: U01 CA179106
Bladder cancer is a common type of cancer that can cause substantial morbidity and mortality among both men and women. Bladder cancer causes over 16,870 deaths per year in the United States. It is estimated that 79,030 new bladder cancer cases will be diagnosed in 2017. Early diagnosis and treatment of these lesions is important to reduce the morbidity, mortality and their attendant costs compared to diagnosis at a later, more symptomatic stage that might involve deep invasion and/or metastasis.
Correct staging of the bladder cancer is crucial for the decision of neoadjuvant chemotherapy treatment and minimizing the risk of under-treatment or over-treatment. Only patients with stage T2 to T4 of muscle-invasive operable urothelial carcinoma of the bladder are recommended for treatment with neoadjuvant chemotherapy. If the response to chemotherapy could be estimated with sufficient accuracy and precision, it would be possible to identify those patients that do not respond, stop the treatment early, and seek alternative treatment. CT is an effective non-invasive modality for measuring primary site gross tumor volume (GTV) and the addition of MRI is on the rise. GTV has been used as a biomarker for predicting treatment outcome of bladder tumors. Other pathological information and diagnostic test (bimanual evaluation, cystoscopy) results and immunohistochemical biomarkers are also useful for staging and treatment response monitoring. Although CT and MRI are promising methods for evaluation of a variety of bladder cancers, the time and costs required for the clinicians to outline cancer margins on a large number of CT and MRI slices for each case makes it difficult to advocate the use of this method for GTV estimation of every patient and of every pre- and post-treatment tumor evaluation.
The goal of this project is to develop a novel multimodality quantitative image analysis tool for bladder cancer (QIBC) to assist radiologists in estimation of GTV and analysis of image characteristics, thereby improving the efficacy of image biomarkers. The QIBC will be designed to use either one or more than one modalities from CT and MRI.
Another goal of this project is to develop novel decision support systems CDSS-S and CDSS-T for bladder cancer staging and for monitoring of bladder cancer treatment response based on multi-modality image-based, pathology-based and immunohistochemical biomarkers. The proposed QIBC, CDSS-S and CDSS-T have the potential to provide non-invasive, objective, and reproducible decision support, thereby reducing the subjectivity and variability in these processes. In order to achieve these goals the team is performing the following specific tasks: (1) to collect a database of multi-modality MR, CT exams of bladder cancers for development, training and testing of the QIBC and CDSS algorithms; (2) to develop advanced computer vision techniques to quantitatively estimate bladder GTV and image characteristics; and (3) to develop predictive models using machine learning techniques to combine multimodality image based, pathological and immunohistochemical biomarkers for cancer staging and determination of non-responders.
In addition, the team plans to design the image analysis and decision support tools in a modular, expandable, and re-trainable framework. The software packages will be versatile and can be adapted to other tumor types or imaging modalities in the future by proper retraining with case samples of the tumor type of interest and expansion of the decision support tools as needed. Therefore, the development of the QIBC, CDSS-S and CDSS-T will potentially benefit not only the bladder tumor patients but also patients with other types of tumors that require staging and monitoring of treatment response.
Link to the MiViewer tool:
https://umich.box.com/shared/static/75ar4bahq6g5f1b0defdcyisxqauw6gc.mp4
References
Hadjiiski L, Weizer AZ, Alva A, Caoili EM, Cohan RH, Cha K, Chan HP. Bladder cancers on CT: preliminary study of treatment response assessment based on computerized volume analysis, WHO and RECIST Criteria. American Journal of Roentgenology 2015; 205(2): 348-352. PMCID: PMC4791536.
Cha K, Hadjiiski L, Samala RK, Chan HP, Caoili EM, Cohan RH. Urinary bladder segmentation in CT urography using deep-learning convolutional neural network and level sets. Med Phys. 2016;43(4): 1882-1896. PMCID: PMC4808067.
Cha K, Hadjiiski L, Samala R K, Chan H-P, Cohan R H, Caoili E M, Paramagul C, Alva A, Weizer AZ. Bladder Cancer Segmentation in CT for Treatment Response Assessment: Application of Deep-Learning Convolution Neural Network. Tomography 2016; 2(4):421-429. PMCID: PMC5241049.
Cha KH, Hadjiiski L, Chan H-P, Weizer AZ, Alva A, Cohan RH, Caoili EM, Paramagul C, Samala RK, “Bladder Cancer Treatment Response Assessment in CT using Radiomics with Deep-Learning.” Scientific Reports 2017 (accepted).
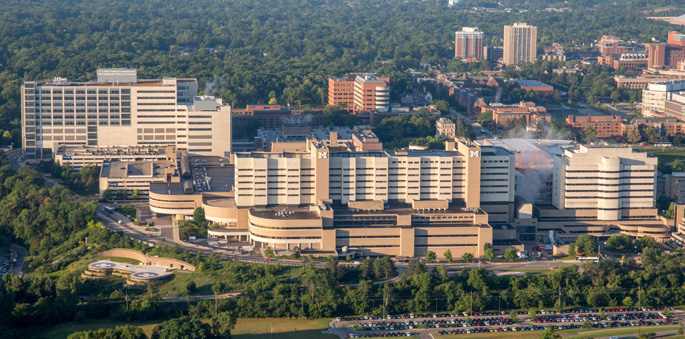