Search QIN

UCLA
Quantitative CT Imaging for Response Assessment when using Dose Reduction Methods
Mike McNitt-Gray, Ph.D.
mmcnittgray@mednet.ucla.edu
Grant Number: U01 CA181156
CT imaging is widely used for assessing response to therapy in clinical practice and in many clinical trials. Recently, there have been tremendous advances in CT hardware (e.g. efficient, lower noise detectors) and software (e.g. iterative reconstruction methods) resulting in scans with greater coverage, thinner reconstructed images and lower radiation dose. Simultaneously, these advances have enabled development of quantitative imaging approaches which range from measures of lesion size (e.g. diameter, volume) to advanced assessments using radiomics features of lesion density, shape, texture, and heterogeneity. As cancer treatments move from conventional chemotherapy to immune and even genetic modification therapies, the need to assess treatment response using features other than lesion size has become increasingly important.
With these advances, there is also an increasing awareness of the substantial impact that acquisition and reconstruction parameters can have on quantitative measures extracted from CT images, depending on the feature being calculated and the parameter being varied. It has been shown that some features are reproducible, but only under a limited set of acquisition and reconstruction conditions (e.g. volumetric measures may be robust to dose level and reconstruction kernel, but not to slice thickness; while shape, edge and texture features can be sensitive to all of these conditions). Thus, while it is desired that the extracted quantitative features should reflect underlying tumor pathophysiology, they may be affected by the image acquisition and reconstruction parameters used, which can mask the true treatment response. This variability may also affect machine learning of diagnostic classifiers, both feature based and convolution neural network based approaches. Therefore, careful and thorough investigations are needed before these are widely deployed.
The overall goal of this work is to assist in the development of robust biomarkers of response using quantitative features extracted from CT imaging. The specific contributions of this work will be to: (1) construct a high throughput pipeline that allows creation of image datasets which represent a wide range of acquisition and reconstruction conditions; (2) use these datasets to evaluate the effects of acquisition and reconstruction conditions on a variety of quantitative imaging tasks and (3) develop strategies that may help extend the range of acquisition and reconstruction conditions over which the desired tasks may be performed. These strategies include: (a) Generating large, augmented datasets with a variety of acquisition and reconstruction parameters for robust machine learning and (b) evaluating specific mitigation strategies such as image denoising methods.
UCLA Computer Vision and Imaging Biomarkers weblink: http://cvib.ucla.edu/
UCLA team QIN Publications
-
Young S, Lo P, Kim G, Brown M, Hoffman J, Hsu W, Wahi-Anwar W, Flores C, Lee G, Noo F, Goldin J, McNitt-Gray M. The effect of radiation dose reduction on computer-aided detection (CAD) performance in a low-dose lung cancer screening population. Med Phys. 2017 Apr;44(4):1337-1346. doi: 10.1002/mp.12128 PMID: 28122122
-
Kalpathy-Cramer J, Mamomov A, Zhao B, Lu L, Cherezov D, Napel S, Echegaray S, Rubin D, McNitt-Gray M, Lo P, Sieren JC, Uthoff J, Dilger SK, Driscoll B, Yeung I, Hadjiiski L, Cha K, Balagurunathan Y, Gillies R, Goldgof D. Radiomics of Lung Nodules: A Multi-Institutional Study of Robustness and Agreement of Quantitative Imaging Features. Tomography. 2016 Dec;2(4):430-437. doi: 10.18383/j.tom.2016.00235. PMID: 28149958
-
Lo P, Young S, Kim HJ, Brown MS, McNitt-Gray MF. Variability in CT lung-nodule quantification: Effects of dose reduction and reconstruction methods on density and texture based features. Med Phys. 2016 Aug;43(8):4854. doi: 10.1118/1.4954845. PMID: 27487903
-
Hoffman J, Young S, Noo F, McNitt-Gray M. Technical Note: FreeCT_wFBP: A robust, efficient, open-source implementation of weighted filtered backprojection for helical, fan-beam CT. Med Phys. 2016 Mar;43(3):1411-20. doi: 10.1118/1.4941953. PMID: 26936725
-
Chong DY, Kim HJ, Lo P, Young S, McNitt-Gray MF, Abtin F, Goldin JG, Brown MS. Robustness-Driven Feature Selection in Classification of Fibrotic Interstitial Lung Disease Patterns in Computed Tomography Using 3D Texture Features. IEEE Trans Med Imaging. 2016 Jan;35(1):144-57. doi: 10.1109/TMI.2015.2459064. PMID: 26208309
-
Young S, Kim HJ, Ko MM, Ko WW, Flores C, McNitt-Gray MF. Variability in CT lung-nodule volumetry: Effects of dose reduction and reconstruction methods. Med Phys. 2015 May;42(5):2679-89. doi: 10.1118/1.4918919. PMID: 25979066
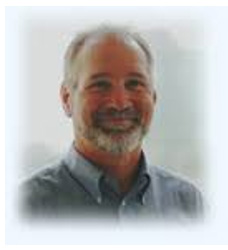