Search QIN

Stanford University Department of Radiology (team#2)
Computing, Optimizing, and Evaluating Quantitative Cancer Imaging Biomarkers
Sandy Napel, Ph.D., Daniel L. Rubin, M.D., M.S.
snapel@stanford.edu; rubin@stanford.edu
Grant Number: U01CA187947
Drs. Napel and Rubin are leading a project, entitled “Computing, Optimizing, and Evaluating Quantitative Cancer Imaging Biomarkers (U01CA187947),” which is developing tools for quantitative analysis of medical images from patients with, or suspected to have, cancer. Quantitative image features that can be computed from medical images are proving to be valuable biomarkers of underlying cancer biology that can be used for assessing response to treatment and predicting clinical outcome. It is now important to discover the best quantitative imaging features for each cancer type and imaging modality that characterize cancers to detect response to new therapeutics, to identify subtypes of cancer, and to correlate with cancer genomics. However, progress is thwarted by the lack of shared software algorithms, architectures, and tools required to compute, compare, evaluate, and disseminate these quantitative imaging features. This project will tackle these challenges by developing and evaluating a publicly available executable and open source software platform, the Quantitative Imaging Feature Pipeline (QIFP), which will give researchers these capabilities for characterizing images of tumors and surrounding tissues for use in multi-center clinical trials and patient monitoring in general. It will also allow researchers to add their own algorithms for computing novel quantitative image features for their own studies, and for building and testing predictive models using these features. In this way, the QIFP will facilitate assessment of the incremental value of new vs. existing feature sets for these purposes.
The QIFP will have the following key attributes that are needed to propel quantitative imaging research forward in the QIN and in the broader research community:
- Web-based, graphical user interface for development of configurable quantitative image feature processing pipelines that will enable researchers to explore combinations of quantitative imaging features
- Expandable and sharable library of quantitative image features algorithms
- Support for a variety of languages for quantitative image feature algorithms, e.g., Matlab, Java, and C/C++, via Docker containers
- Connectivity to images and other data stored in
- the Cancer Imaging Archive (TCIA)
- ePAD systems (another QIN project for image annotation/curation)
- local data stores
- PACS systems via DICOM
- Cloud-based cache of data and software
- Machine learning algorithms that permit researchers to efficiently establish how well a quantitative image feature or combination of features predicts a clinical or molecular variable.
The QIFP will fill a substantial gap in the cancer imaging community by providing the tools and infrastructure to assess the value of novel quantitative imaging features of cancer, and thereby accelerate incorporation of new imaging biomarkers into single- and multi-center clinical trials. It will also have additional impact by providing a means to disseminate and to promote the use of the quantitative imaging to the broader community.
Stanford team’s lab URL: http://med.stanford.edu/riipl.html
References:
-
S. Echegaray, S. Bakr, D. L. Rubin, S. Napel, “Quantitative Image Feature Engine (QIFE): An open-source, modular engine for 3D quantitative feature extraction from volumetric medical images,” J Digit Imaging, 2017 Oct 6 doi: 10.1007/s10278-017-0019-x. [Epub ahead of print] PMID: 28993897.
-
J. Wu, G. Cao, X. Sun, J. Lee, D.L. Rubin, S. Napel, A.W. Kurian, B. Daniel, R. Li, “Intratumoral spatial heterogeneity by perfusion MR imaging predicts recurrence-free survival in locally advanced breast cancer treated with neoadjuvant chemotherapy,” Radiology 288(1):26-35, 2018 doi: 10.1148/radiol.2018172462.
-
Y. Balagurunathan, A. Beers, J. Kalpathy-Cramer, M. McNitt-Gray, L. Hadjiiski, B Zhao, J. Zhu, H. Yang, S.F. Yip, H. J.W.L. Aerts, S. Napel, D. Cherezov, K. Cha, H-P Chan, C. Flores, A. Garcia, R. Gillies, D. Goldgof, “Semi-Automated Pulmonary Nodule Interval Segmentation using the NLST Data,” Med Phys 2018; 45:1093-1107. PMID:29363773. PMCID: PMC5952359.
-
W. Zhang, G. Bouchard, A. Yu, M. Shafiq, M. Jamali, J. Shrager, K. Ayers, S. Bakr, A. Gentles, M. Diehn, A. Quon, R. West, V. S. Nair, M. van de Rijn, S. Napel, S. K. Plevritis, “Metabolic reprogramming in human lung adenocarcinoma mediated by GFPT2-expressing cancer-associated fibroblasts is prognostically significant,” Cancer Res., 78(13):3445-3457 July 2018. PMID: 29760045. PMCID: PMC6030462.
-
O. Bernard, A. Lalande, C. Zotti, F. Cervenansky, X. Yang, P-A. Heng, I. Cetin, K. Lekadir, O. Camara, M. Angel Gonzales Ballester, G. Sanroma, S. Napel, S. Petersen, G. Tziritas, E. Grinias, M. Khened, V. A. Kollerathu, G. Krishnamurthi, M-M. Rohé, X. Pennec, M. Sermesant, F. Isensee, P. Jäger, K. H. Maier-Hein, C. F. Baumgartner, L. M. Koch, J. M. Wolterink, I. Išgum, Ye. Jang, Y. Hong, J. Patravali, S. Jain, O. Humbert, P-M. Jodoin, “Deep Learning Techniques for Automatic MRI Cardiac Multi-structures Segmentation and Diagnosis: Is the Problem Solved?” doi: 10.1109/TMI.2018.2837502. [Epub ahead of print] May 2018.
-
S. Napel, W. Mu, B. Jardim-Perassi, H. J. W. L. Aerts, R. Gillies, “Quantitative Imaging of Cancer in the Post-genomic Era: Radio(geno)mics, Deep Learning and Habitats,” in press, Cancer Research, July 2018.
-
S. Bakr, O. Gevaert, S. Echegaray, K. Ayers, M. Zhou, M. Shafiq, H. Zheng, W. Zhang, A.N.C. Leung M. Kadoch, J. Shrager, A. Quon, D.L. Rubin, S. K. Plevritis*, S. Napel*, “A Radiogenomic Dataset of Non-Small Cell Lung Cancer,” in press, Nature Scientific Data, July 2018.
-
J. Wu, X. Li, X. Teng, D. L. Rubin, S. Napel, B. L. Daniel, R. Li, “MR imaging and molecular features associated with tumor infiltrating lymphocytes in breast cancer,” in press, Breast Cancer Research, August 2018.
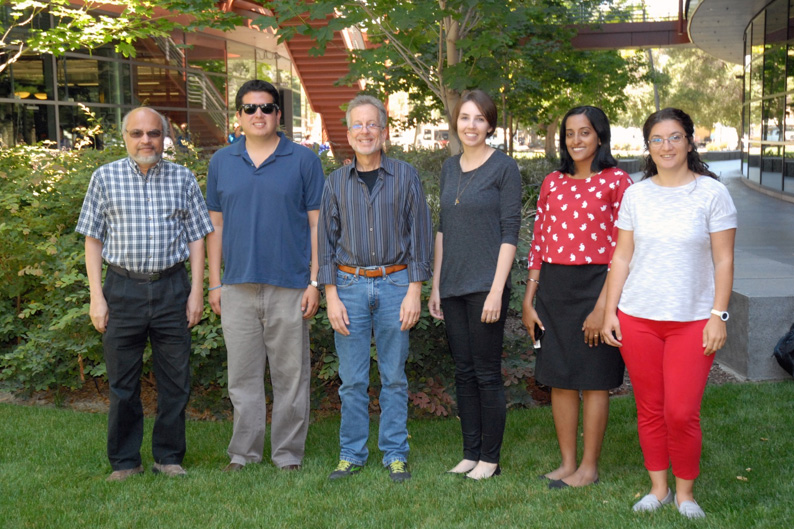