Search QIN

Massachusetts General Hospital
Quantitative MRI of Glioblastoma Response
Jayashree Kalpathy-Cramer, Ph.D
kalpathy@nmr.mgh.harvard.edu
Grant Number: U01 CA154601
The goal of the MGH Quantitative Imaging Network (QIN) project is to develop quantitative imaging tools to facilitate clinical decision making, particularly in the setting of treatment of glioblastoma (GBM). This is in harmony with the team’s overall research goals of using mechanistic imaging to develop biomarkers of therapeutic response. MGH seeks to improve the quantitative performance of MRI methods including perfusion, permeability and diffusion MRI through advances in acquisition and to facilitate their use in the clinic through the development of open-source, user friendly tools for image analysis.
Simultaneous-multiple-slice DSC-MRI: Improved slice coverage of gradient echo spin echo (GESE) sequences for dynamic susceptibility contrast (DSC) MRI was achieved using a simultaneous-multiple-slice (SMS) method. The developed SMS sequence doubled the slice coverage while retaining excellent SNR retention. The SMS DSC sequence has been incorporated into research protocols and is available through Siemens.
Vessel Architecture Imaging (VAI): VAI, an extension of vessel caliber imaging, has been shown to be an early biomarker of treatment response in GBM patients on anti-angiogenic therapies. VAI exploits the temporal differences between the GE and SE signals by examining the difference in the peak positions of these signals and provide insights into the microcirculation and oxygenation patterns. Improvements in microcirculation identified by the VAI were predictive of improved survival.
Quantitative imaging pipeline for GBM: MGH has developed a robust image analysis pipeline for within-patient and longitudinal registration for both structural images (T1 pre and post contrast, T2, FLAIR) as well as parametric maps. Tools for automatic tumor segmentation using deep learning have been developed and are being validated in retrospective and prospective trials. Tumor growth models have also been developed and their use in adaptive radiotherapy is being evaluated.
Radiomics: The MGH team has developed a robust radiomics pipeline for the extraction of imaging features from CT or MR images given a Region of Interest (ROI). This pipeline includes features related to shape, size, texture, margins and local and global shape descriptors.
Deep Learning: Deep learning based methods are being used as “virtual biopsies” to recognize patterns directly from imaging. These including the prediction of IDH status from MR imaging in multi-institutional data. Deep learning is also being used to separate tumor recurrence from pseudo-progression, identify tumor grade and predict survival.
Clinical applications: The developed acquisition protocols and imaging analysis methods are being applied to data from several clinical trials in patients with glioblastoma (GBM) and primary CNS lymphoma patients.
Deliverables: Tools for T1 mapping, DSC and DCE analysis to the community as open-source software packages, both stand-alone and as 3D Slicer modules. Data is being shared through the Cancer Imaging Archive (TCIA). The SMS sequences are available to the community through Siemens. The entire open-source image analysis pipeline available as a Docker module. The radiomics pipeline is also publicly available as an easily installable Python package.
Publications:
-
Elhalawani H, Mohamed ASR, White AL, Zafereo J, Wong AJ, Berends JE, AboHashem S, Williams B, Aymard JM, Kanwar A, Perni S, Rock CD, Cooksey L, Campbell S, Ding Y, Lai SY, Marai EG, Vock D, Canahuate GM, Freymann J, Farahani K, Kalpathy-Cramer J, Fuller CD., Matched computed tomography segmentation and demographic data for oropharyngeal cancer radiomics challenges., Sci Data. 2017 Jul 4;4:170077. PMID: 28675381
-
Kalpathy-Cramer J, Zhao B, Goldgof D, Gu Y, Wang X, Yang H, Tan Y, Gillies R, Napel S., A Comparison of Lung Nodule Segmentation Algorithms: Methods and Results from a Multi-institutional Study. J Digit Imaging. 2016 Aug;29(4):476-87, PMID: 26847203
-
Hansen MB, Tietze A, Kalpathy-Cramer J, Gerstner ER, Batchelor TT, Østergaard L, Mouridsen K., Reliable estimation of microvascular flow patterns in patients with disrupted blood-brain barrier using dynamic susceptibility contrast MRI, J Magn Reson Imaging. 2016 Nov 30. [Epub ahead of print] PMID: 27902858
-
Kalpathy-Cramer J, Chandra V, Da X, Ou Y, Emblem KE, Muzikansky A, Cai X, Douw L, Evans JG, Dietrich J, Chi AS, Wen PY, Stufflebeam S, Rosen B, Duda DG, Jain RK, Batchelor TT, Gerstner ER., Phase II study of tivozanib, an oral VEGFR inhibitor, in patients with recurrent glioblastoma, J Neurooncol. 2016 Nov 16. [Epub ahead of print], PMID: 27853960
-
Le M, Delingette H, Kalpathy-Cramer J, Gerstner E, Batchelor T, Unkelbach J, Ayache N., MRI Based Bayesian Personalization of a Tumor Growth Model., IEEE Trans Med Imaging. 2016 Apr 29. [Epub ahead of print], PMID: 28113549
-
Farahani K, Kalpathy-Cramer J, Chenevert TL, Rubin DL, Sunderland JJ, Nordstrom RJ, Buatti J, Hylton N, Computational challenges and Collaborative projects in the NCI Quantitative Imaging Network, Tomography, Tomography. 2016 Dec;2(4):242-249.
-
Kalpathy-Cramer J, Mamomov A, Zhao B, Lu L, Cherezov D, Napel S, Echegaray S, Rubin D, McNitt-Gray M, Lo P, Sieren JC, Uthoff J, Dilger SK, Driscoll B, Yeung I, Hadjiiski L, Cha K, Balagurunathan Y, Gillies R, Goldgof D., Radiomics of Lung Nodules: A Multi-Institutional Study of Robustness and Agreement of Quantitative Imaging Features., Tomography. 2016 Dec;2(4):430-437. PMID: 28149958
-
Gerstner ER1, Zhang Z2, Fink JR3, Muzi M3, Hanna L2, Greco E2, Prah M4, Schmainda KM4, Mintz A5, Kostakoglu L6, Eikman EA7, Ellingson BM8, Ratai EM9, Sorensen AG10, Barboriak DP11, Mankoff DA12; ACRIN 6684 Trial Group., ACRIN 6684: Assessment of Tumor Hypoxia in Newly Diagnosed Glioblastoma Using 18F-FMISO PET and MRI., Clin Cancer Res. 2016 Oct 15;22(20):5079-5086. Epub 2016 May 16., PMID: 27185374
-
Kurland BF, Aggarwal S, Yankeelov TE, Gerstner ER, Mountz JM, Linden HM, Jones EF, Bodeker KL, Buatti JM., Accrual Patterns for Clinical Studies Involving Quantitative Imaging: Results of an NCI Quantitative Imaging Network (QIN) Survey.Tomography. 2016 Dec;2(4):276-282. PMID: 28127586
-
Kalpathy-Cramer J, Gerstner ER, Emblem KE, Andronesi OC, Rosen B., Advanced magnetic resonance imaging of the physical processes in human glioblastoma. Cancer Res. 2014 Sep 1;74(17):4622-37. PMID: 25183787
Laboratory website:
https://www.martinos.org/lab/qtim
Code:
https://github.com/QTIM-Lab
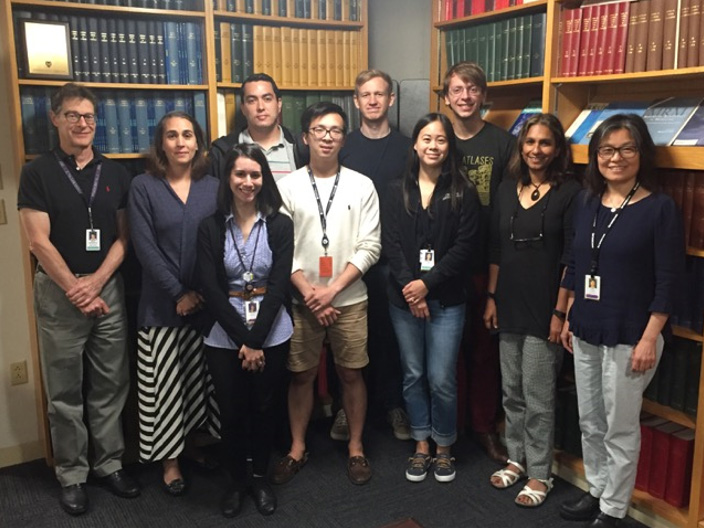