Search QIN

Columbia University Medical Center
Quantitative Volume and Density Response Assessment: Sarcoma and HCC Model
Lawrence H Schwartz, M.D., Binsheng Zhao, D.Sc.
lschwartz@columbia.edu; bz2166@cumc.columbia.edu
Grant Number: U01 CA140207
Current methods for assessing patient response to cancer treatments are outdated. The standard Response Evaluation Criteria in Solid Tumor (RECIST) is a unidimensional measurement that accurately quantifies change in total tumor burden only when tumors are spherical and change size in a spherical fashion, which is often not the case. RECIST discards information about changes in tumor volume and composition, which are readily quantifiable from CT and can capture the more complex changes in tumors caused by modern therapies, especially targeted therapies. Cut-off values for categorizing response and progression with unidimensional measurements were determined by physical palpation, the standard clinical practice 30 years ago. Despite decades of remarkable improvements in medical imaging and image analysis algorithms, response assessment methods have remained almost unchanged. A critical unmet need in new drug development and clinical evaluation is to identify and validate new criteria for assessment of response to novel targeted therapies.
The goal of this research is to develop new response assessments for cancer treatment based on CT imaging of changes in tumor volume and necrosis fraction. This study will seek a proof of concept using two types of tumors in which RECIST is known to correlate poorly with tumor response to treatment and clinical outcome. HCC is one of the most common malignancies worldwide, and sarcomas, though rare, carry the same molecular alterations as many other heterogeneous cancers and are the classic cancer studied in drug discovery. Specific Aim 1 will demonstrate that assistance from new automated segmentation algorithms can reduce the variability in radiologists’ measurement of lung, liver, and lymph tumors. This task will use images from 276 patients already collected by SARC 011, a large phase II multicenter clinical trial of sarcoma. Aim 2 will correlate tumor volume and necrosis fraction with clinical outcome in SARC 011 and CALGB 80802, a phase III trial of HCC with an estimated enrollment of 480 patients. The proposed research will first develop criteria based on quantitative biomarkers (tumor volume and necrosis fraction) and then compare the predictive value of these criteria to the current clinical standard using a concordance probability estimate. Aim 3 will explore the correlation of these criteria to other biochemical markers, and use a concordance probability estimate to determine whether the combination of imaging biomarkers with biochemical markers affords superior prediction of patient survival as compared to either alone. Aim3 will use data from three companion biology studies of SARC 011 and CALGB 80802. Development and validation of the new criteria will have substantial health significance because evidence that volume and necrosis changes are early biomarkers of response or progression will guide clinical trials and patient treatment. The new criteria will be widely applicable to clinical practice since CT is the most common imaging modality for cancer, the new algorithms run on popular imaging platforms, and this method will reduce the time required by radiologists.
Laboratory URL: https://www.columbiaradiology.org/cialab
Recent published papers
- Beichel R, Smith B, Bauer C, Ulrich E, Ahmadvand P, Budzevich M, Gillies R, Goldgof D, Grkovski M, Hamarneh G, Huang Q, Kinahan P, Laymon C, Mountz J, Muzi J, Muzi M, Sadek N, Oborski M, Tan Y, Zhao B, Sunderland J, and Buatti J. Multi-site Quality and Variability Analysis of 3D FDG PET Segmentations based on Phantom and Clinical Image Data. Med Phys. 2017; 44(2):479-496. PMID: 28205306
- Zhao B, Tan Y, Qi J, Xie C, Tsai W-Y, Schwartz LH. Reproducibility of radiomics for deciphering tumor phenotype with imaging. Nat Sci Rep6; 23428, 2016. doi:10.1038/srep23428. PMID: 27009765
- Yang H, Schwartz LH, and Zhao B. A Response Assessment Platform for Development and Validation of Imaging Biomarkers in Oncology. Tomography. 2016; 2(4):406-410. (PMID not yet available)
- Koshkin VS, Bolejack V, Schuetze S, Schwartz LH, Zhao B, Chugh R, Wahl RL, Reinke DL, Helman LJ, Patel S, Baker LH. The WHO and What of Imaging in Sarcoma and Correlation With Survival. JCO 2016; 34(30): 3680-5. PMID: 27573658
- Yankeelov TE, Mankoff DA, Schwartz LH, Lieberman F, Buatti J, Mountz JM, Erickson B, Fennessy F, Huang W, Kalpathy-Cramer J, Wahl R, Linden H, Zhao B, Rubin D, Quantitative Imaging in Cancer Clinical Trials. Clin Cancer Res 2016 Jan 15;22(2):284-90. PMID: 26773162
- Kalpathy-Cramer J, Mamomov A, Zhao B, Lu L, Cherezov D, Napel S, Echegaray S, Rubin D, McNitt-Gray M, Lo P, Sieren JC, Uthoff J, Dilger SKN, Driscoll B, Yeung I, Hadjiiski L, Cha K, Balagurunathan Y, Robert Gillies R, and Goldgof D. Radiomics of lung nodules: a multi-institutional study of robustness and agreement of quantitative imaging features. Tomography. 2016; 2(4):430-437. PMID: 28149958
- Kalpathy-Cramer J, Zhao B, Goldgof D, Gu Y, Wang X, Yang H, Tan Y, Gillies R, Napel S, A Comparison of Lung Nodule Segmentation Algorithms: Methods and Results from a Multi-institutional Study. J Digit Imaging 2016; 29(4):476-87. PMID: 28149958
- Yan J, Schwartz LH, and Zhao B. Semi-automatic segmentation of liver metastases on volumetric CT images. Med Phys. 2015 Nov;42(11):6283. PMID: 26520721
- Zhao B, Lee S, Lee HJ, Tan Y, Qi J, Persigehl T, Mozley PD and Schwartz LH. Variability in assessing treatment response: metastatic colorectal cancer as a paradigm. Clin Cancer Res. 2014; 20(13):3560-8. PMID: 24780294
- Tan Y, Schwartz LH and Zhao B, Segmentation of lung tumors on CT Scans using Watershed and Active Contours. Med Phys. 2013; 40(4):043502. PMID: 23556926
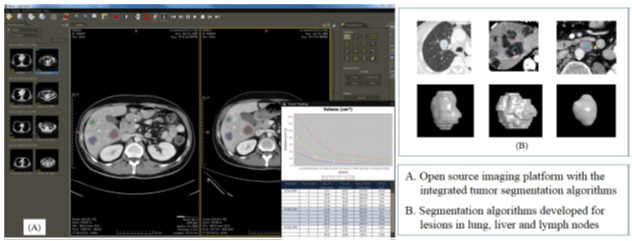